This is the decade for data transformation. The key is to ensure that data is available for driving critical business decisions. The capabilities that an organization will absolutely need are:
- Data as a product where teams can access the data instantly and securely
- Data sovereignty where the governance policies and processes are well understood and implemented by a combination of people, process and tools
- Data as a prized asset in the organization – Data maintenance with high quality, consistency, and trust
The significance of data to run an enterprise business is critical. Data is recognized to be a major factor in driving informed business decisions and providing optimal service to clients. Hence, it is crucial to have good quality data and an optimized approach to DQM.
As the volume of data in an organization increases exponentially, manual DQM becomes challenging. Data that flows into an organization is of high volume, mostly real-time and may change characteristics. Advanced tools and modern technology are needed to provide the automation that would drive accuracy and speed to achieve the desired level of data quality for an organization.
DQM in an enterprise is a continuous journey. To be relevant for the business, a regular flow of learning needs to be fed into the process. This is important for improving the results as well as for adapting to the changing nature of data in the enterprise. A continuous process of assessment of data quality, implementation of rules, data remediation and learning feedback is necessary to run a successful DQM program.
The process of DQM can be depicted with the help of the following diagram –
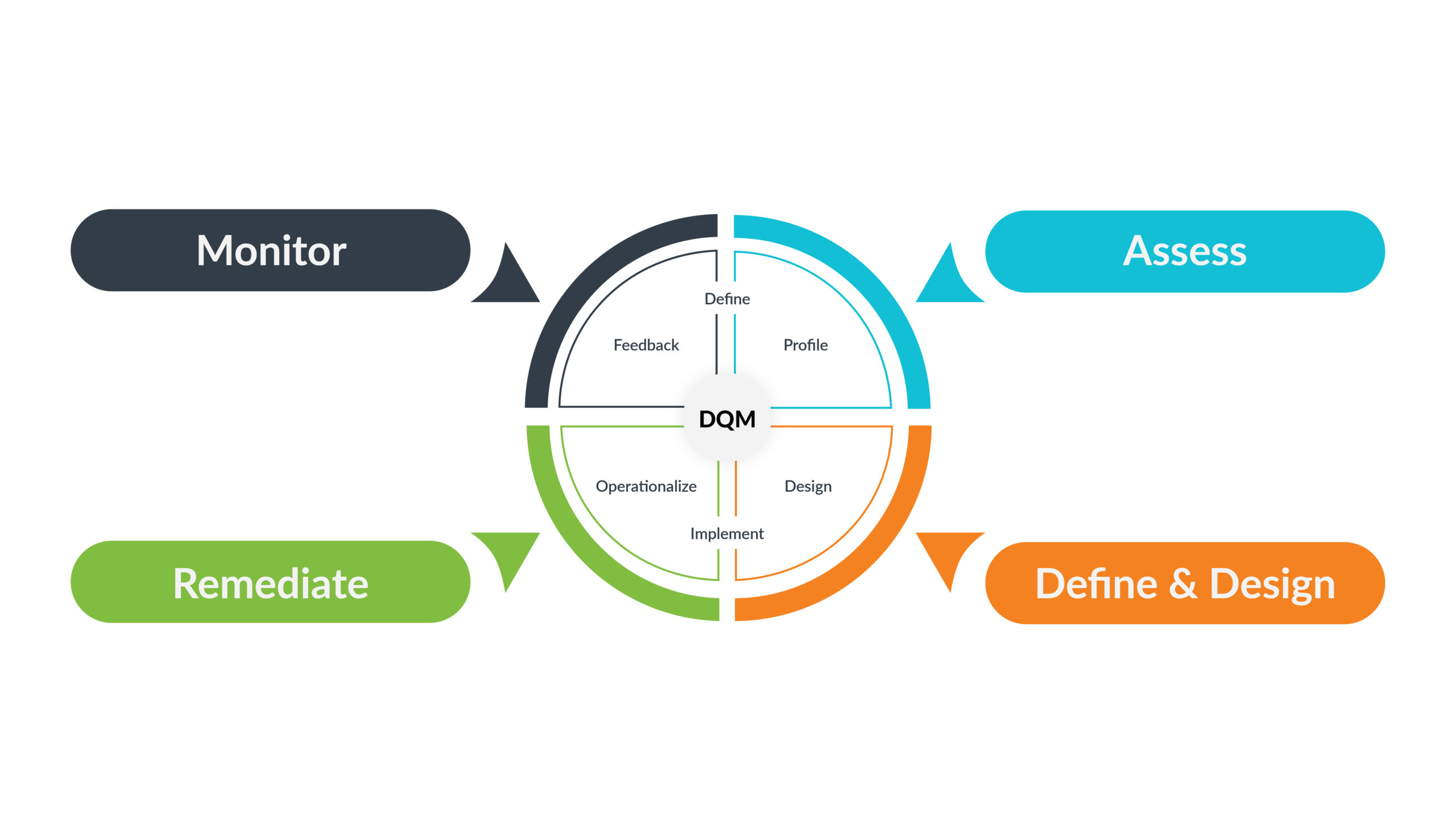
How does Machine Learning (ML) help in DQM?
To drive these capabilities and accelerate data transformation for an organization, it is extremely important to have a strong DQM strategy. The burden of DQM needs to shift from manual mode to a more agile, scalable and automated process. The time-to-value for an organization’s DQM investments should be minimized.
Focusing on innovation, not infrastructure, is how businesses can get value from data and differentiate themselves from their competitors. More than ever, time, and how it’s spent, is perhaps a company’s most valuable asset. Business and IT teams today need to be spending their time driving innovation, and not spending hours on manual tasks.
By taking over DQM tasks that have traditionally been more manual, an ML-driven solution can streamline the process in an efficient cost-effective manner. Since an ML-based solution can learn more about an organization and its data, it can make more intelligent decisions about the data it manages, with minimal human intervention. The nature of data in an organization is also ever changing. DQM rules need to constantly adapt to such changes. ML-driven automation can be applied to greatly automate and enhance all the dimensions of Data Quality, to ensure speed and accuracy for small to gigantic data volumes.
The application of ML in different DQ dimensions can be articulated as below:
- Accuracy: Automated data correction based on business rules
- Completeness: Automated addition of missing values
- Consistency: Delivery of consistent data across the organization without manual errors
- Timeliness: Ingesting and federating large volumes of data at scale
- Validity: Flagging inaccurate data based on business rules
- Uniqueness: Matching data with existing data sets, and remove duplicate data
Fresh Gravity’s Approach to Data Quality Management
Our team has a deep and varied experience in Data Management and comes with an efficient and innovative approach to effectively help in an organization’s DQM process. Fresh Gravity can help with defining the right strategy and roadmap to achieve an organization’s Data Transformation goals.
One of the solutions that we have developed at Fresh Gravity is DOMAQ (Data Observability, Monitoring and Data Quality Engine), which enables business users, data analysts, data engineers, and data architects to detect, predict, prevent, and resolve issues, sometimes in an automated fashion, that would otherwise break production analytics and AI. It takes the load off the enterprise data team by ensuring that the data is constantly monitored, data anomalies are automatically detected, and future data issues are proactively predicted without any manual intervention. This comprehensive data observability, monitoring, and data quality tool is built to ensure optimum scalability and uses AI/ML algorithms extensively for accuracy and efficiency. DOMAQ proves to be a game-changer when used in conjunction with an enterprise’s data management projects (MDM, Data Lake and Data Warehouse Implementations).
To learn more about the tool, click here .
For a demo of the tool or for more information about Fresh Gravity’s approach to Data Quality Management, please write to us at soumen.chakraborty@freshgravity.com, vaibhav.sathe@freshgravity.com or sudarsana.roychoudhury@freshgravity.com .
Leave a Reply